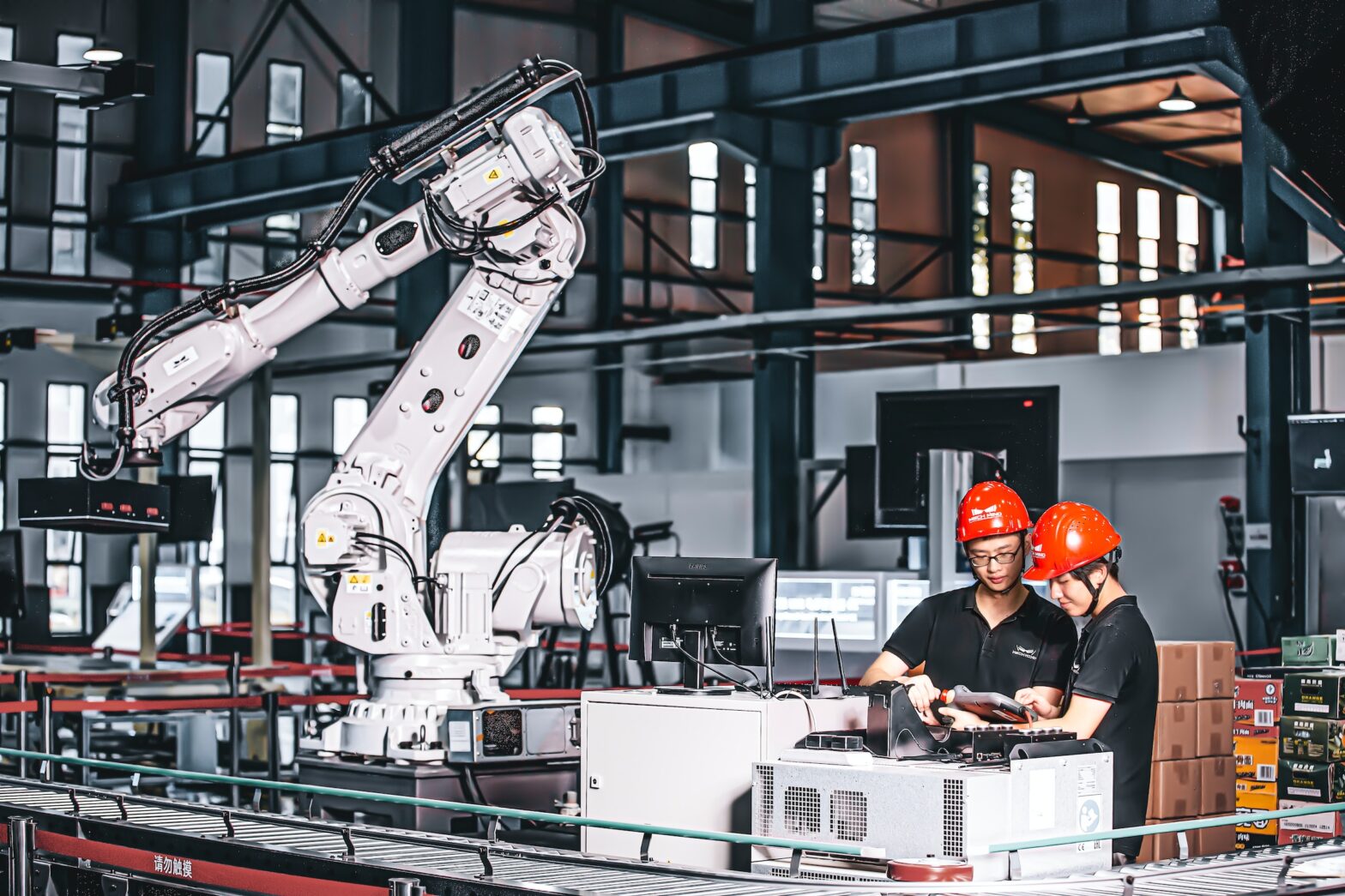
Industry 5.0 Manufacturing Best Practices
Prepare for Industry 5.0 manufacturing with sustainable practices, human-machine collaboration, and an agile manufacturing approach.
8 minute read
•Manufacturing
Bookmark me
|Share on
Edge computing combined with AI is supporting more intelligent Internet of Things (IoT) devices—a paradigm shift called “edge intelligence.”
Artificial intelligence (AI) is growing in relevance within our everyday lives. In addition to popularly available applications like OpenAI’s ChatGPT, AI-driven technologies are driving innovations in art, internet and website search, customer service interactions, and gaming, with the potential to impact virtually any consumer environment featuring internet connectivity.
Meanwhile, AI already has a strong foothold in the business world. In addition to centralized, cloud-based AI applications like advanced analytics, business leaders are looking for more AI use cases beyond the data center—that is, closer to the edge of operations and computing, where equipment, sensors, and user interfaces reside.
That’s why alongside AI, “we have witnessed IT architectures maturing and adopting edge technologies, leading to bold proclamations that the ‘edge is eating the world!’” as Forbes describes.
In this article, we investigate the convergence of edge computing and IoT, and how it’s facilitating AI applications closer to business end-users, equipment, and devices. We consider the advantages, opportunities, and challenges of edge intelligence. Finally, we consider future trends and use cases as this inevitable development matures.
The cloud already supports countless IoT-driven benefits. “High-performance computing, a storage infrastructure, processing, and analysis of large-scale data [give] IoT the opportunity to be robust, intelligent, and self-configuring,” according to an October 2023 IEEE World Forum discussion. Cloud also supports edge computing applications, where data is collected, processed, and analyzed at the network’s edge (versus a central point).
But there are limitations in terms of how the cloud can service equipment, users, and devices at the edge. As the need for complex computing grows at these edge locations, “there is a need to improve IoT devices and reduce these bottlenecks largely caused by moving huge chunks of data from the IoT devices to data centers for processing and analysis.,” according to TechRepublic. “IoT needs stable connectivity to be super-efficient, and edge computing guarantees that.”
Pushing AI applications closer to the edge requires stable connectivity and high computing power. Consider the following scenario: a factory wants to implement AI algorithms in its machines so they can make real-time decisions within milliseconds. This is impossible if their data is transported to a remote server for processing and then back to the machine. With edge computing, these processes can now happen on the device itself.
Now, the edge computing market is poised to grow roughly $155.9 billion by 2030 with a CAGR of 38.9%, Bloomberg reports, where “the integration of artificial intelligence (AI) into the edge environment is expected to propel market expansion.”
As edge computing and IoT converge to support new AI applications, there are dozens of opportunities that arise for B2B businesses, as well as B2C companies and the consumers they service. Some of the key benefits include:
Lower Latency: Edge Intelligence enables faster decision-making at the device level, reducing latency and improving response time. This is especially important in scenarios where decisions need to be made quickly, such as industrial automation of robots and machines.
Reduced Network Traffic: With AI applications running on devices themselves, there is less need for data to be transmitted back and forth to a central server, reducing network traffic and freeing up bandwidth.
Improved Security: Edge Intelligence allows for more secure processing of data as it is being done locally on the device, rather than sending sensitive information back and forth over networks.
Cost Savings: With edge computing and AI applications working together, there is potential for cost savings in terms of reduced infrastructure needs and energy consumption.
Contextualized Decision Support: Users at the edge can access real-time decision support and insights, resulting in more efficient and effective decision-making in their unique roles and contexts. For example, a bank branch can achieve localized analytics and AI-driven recommendations without burdening their companies’ centralized cloud computing resources.
While there are numerous opportunities for edge intelligence, there are also challenges that need to be addressed. These include:
Data Management: With more data being generated and processed at the edge, proper data management practices need to be put in place to ensure data is properly collected, stored, and analyzed.
Device Limitations: Edge devices often have limited computing power, storage, and bandwidth capabilities. This means AI applications need to be optimized to work within these constraints; or, organizations can prioritize more powerful edge devices.
Integration Complexity: As edge intelligence becomes increasingly integrated with IoT and cloud solutions, there is the potential for increased complexity in implementation and management.
Orchestrating a “Computing Continuum”: With AI applications now being deployed at multiple points in the computing continuum (device to cloud and vice versa), there is a need for orchestration and coordination between these different layers to ensure seamless operation.
As edge intelligence continues to mature and evolve, we can expect to see a wide range of use cases and applications. Some potential trends include:
Industrial Automation: With edge intelligence, machines can make real-time decisions without relying on a central server. Since edge AI can incorporate any quantity and variety of data, machines can employ a wider variety of sensors and functions to create a more accurate picture of their environments.
Hyper-Personalized Healthcare: With the rise of wearables and remote medical monitoring, edge computing will play an important role in collecting and analyzing patient data in real time, potentially improving diagnosis and treatments.
Smart Cities: Edge intelligence can help power smart cities by enabling real-time data analysis and decision-making for things like traffic control, energy management, and waste management.
Decarbonization: By leveraging machine learning algorithms, edge intelligence can help optimize energy consumption and reduce carbon emissions.
As these trends continue to unfold, the convergence of edge computing and IoT will only become more prevalent in our daily lives. Businesses that leverage these technologies and stay ahead of the curve will be well-positioned for success in the future.
It’s essential to keep an eye on emerging trends and use cases in this space in order to stay competitive. Here we share four steps you can take today to begin preparing your organization for edge intelligence use cases, no matter the industry in which your organization operates.
Understand Your Business Needs: Evaluate which processes and decisions within your organization could benefit from faster, more accurate data analysis. Identify areas where edge intelligence can improve efficiency and productivity.
Assess Data Management Capabilities: With the growth of edge computing, there will be an increase in IoT devices generating vast amounts of data. Organizations should assess their current data management capabilities and ensure they have the infrastructure and processes in place to handle this influx of data.
Leverage Partnerships: As edge intelligence continues to evolve, it’s essential to form partnerships with providers who can offer expertise and solutions in the space, as well as access to cutting-edge technology.
Ensure Security Measures: With more data being generated and processed at the edge, it’s crucial to have strong security measures in place. This includes protecting data both in transit and at rest on edge devices.
By taking these steps, your organization can start preparing for the convergence of edge computing and IoT, setting the stage for future success.
Already, “programmable AI at the edge and edge-as-a-service market models with API and developer-first approaches are beginning to democratize this space,” as Forbes describes. Much like the cloud technology revolution, edge intelligence will become increasingly accessible and user-friendly, opening up the potential for widespread deployment in various industries. This democratization will allow organizations of all sizes to leverage edge computing and AI applications to improve processes, increase efficiency, and drive innovation.
Uvation supports global enterprises as they unlock the full potential of edge intelligence. Our team of experts can help you poise your organization for growth and efficiency, and create custom solutions to meet your unique business needs. Contact us today to learn more about how we can help you harness edge intelligence for success.
Bookmark me
|Share on
Prepare for Industry 5.0 manufacturing with sustainable practices, human-machine collaboration, and an agile manufacturing approach.
8 minute read
•Manufacturing
5G and edge computing are no longer simply technology trends. They have the potential to revolutionize the way businesses operate.
8 minute read
•Manufacturing