As AI rapidly integrates into your enterprise—from streamlining decision-making to powering advanced customer insights—having the right GPU infrastructure becomes crucial. Large language models (LLMs) like GPT-4 and multimodal systems demand GPUs that not only handle massive computational tasks but also scale efficiently as workloads grow. Choosing the right setup is key to ensuring both performance and cost-effectiveness.
Dual-GPU configurations offer a practical solution by leveraging two GPUs to share computational tasks. This setup accelerates training times and reduces inference latency, which is critical for businesses like yours, where speed and efficiency directly impact competitive advantage. But when it comes to selecting between NVIDIA’s H100 and H200 NVL, the decision goes beyond just raw performance—it’s about how each option fits your long-term strategy.
The H100 is a proven solution, offering reliable performance at a more accessible price point. It’s ideal if you’re looking to enhance existing AI workloads without overspending. However, as you scale up and tackle more complex models, the H200 NVL becomes a compelling choice. With enhanced memory bandwidth and next-gen features like improved NVLink connectivity, the H200 NVL is designed for businesses aiming to stay ahead in the rapidly evolving AI landscape.
Now, let’s break down the factors that matter most in your decision. From upfront costs to energy efficiency and scalability, we’ll explore what makes the H100 and H200 NVL distinct, helping you assess which delivers the best ROI for your AI infrastructure.
2. Understanding Dual-GPU Configurations
What Is a Dual-GPU Setup?
In a dual-GPU configuration, you use two GPUs within a single system, working together via high-speed connections like NVIDIA’s NVLink. This setup effectively doubles your available computational power, allowing for faster model training and improved inference times. For your enterprise, this means increased efficiency and the ability to handle larger datasets and more complex models without significant delays.
Benefits for Your Enterprise AI
The real value of dual-GPU setups becomes clear when you consider how they can accelerate both training and inference. With two GPUs working together, you reduce processing times for tasks such as training deep learning models or running real-time predictions. This speed not only improves productivity but also helps you bring AI-driven products and services to market faster, which gives you a competitive advantage.
Another significant benefit is scalability. As your AI models grow larger and more intricate, dual-GPU systems ensure you can meet these increasing demands without sacrificing performance. For example, training large language models (LLMs) requires more computational power than a single GPU can handle. A dual-GPU setup, like the H100 or H200 NVL, allows you to scale your operations and handle even the most complex models with ease.
However, there are trade-offs to consider. While dual-GPU systems offer substantial performance gains, they also require a higher initial investment and more power. Balancing the cost against the performance improvements is essential for ensuring that your investment pays off in the long term.
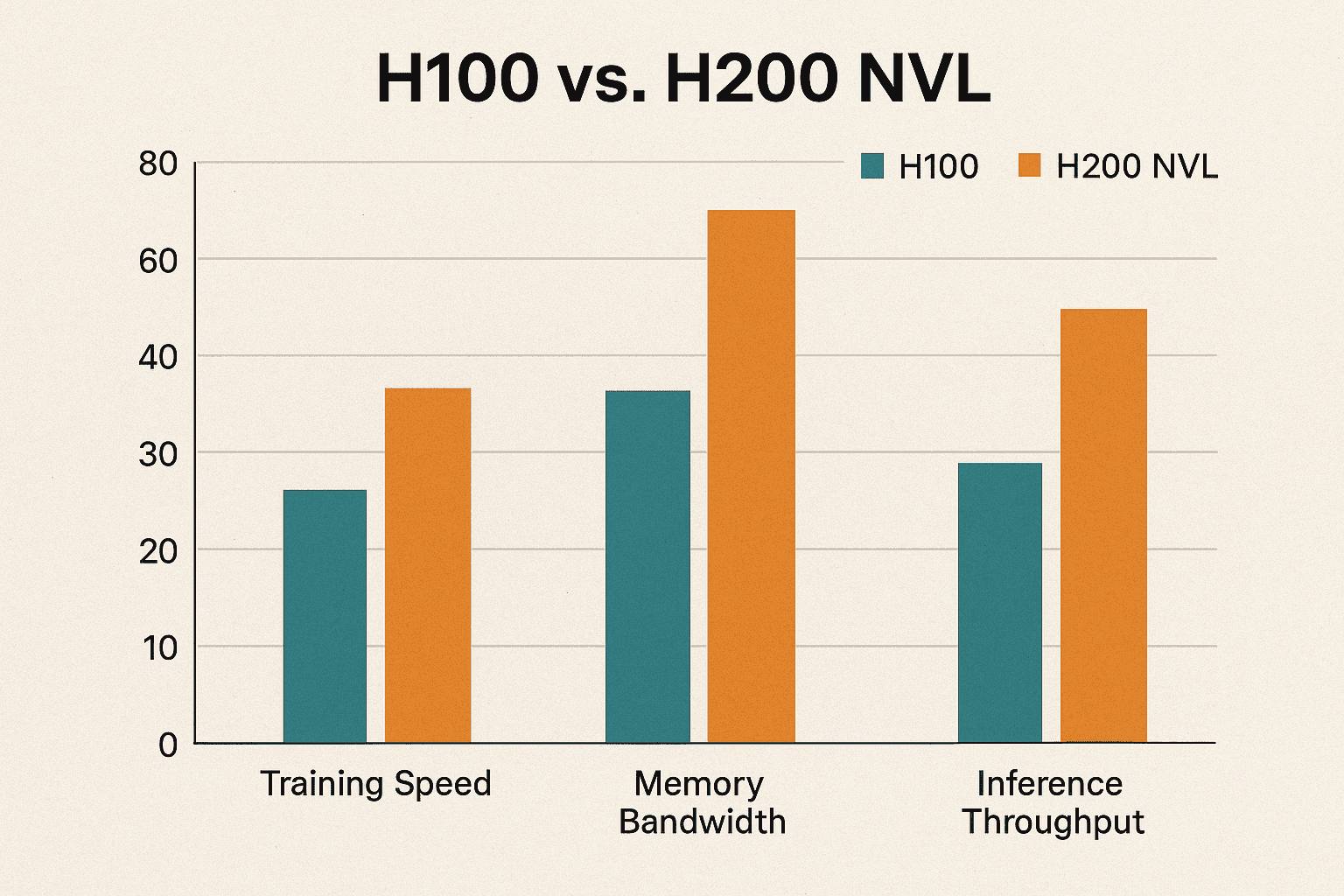
Factors Impacting Your ROI
When it comes to ROI, the benefits of a dual-GPU setup extend beyond raw performance. The upfront cost is a key consideration, as you’ll need to invest in not just the GPUs but also compatible servers, NVLink bridges, and effective cooling solutions. While the H100 offers a more affordable starting point, the H200 NVL—although more expensive—provides enhanced scalability and long-term value for enterprises planning to expand their AI workloads.
Energy consumption is another critical factor. Dual-GPU systems naturally draw more power, which can lead to higher operational costs. But the efficiency of the GPUs will determine how much you spend on energy over time. As you scale your infrastructure, considering performance per watt will be vital to minimizing long-term expenses.
Additionally, the integration of dual-GPU setups into your existing infrastructure may require some adjustments. Optimizing software for multi-GPU systems and ensuring compatibility with legacy tools can present challenges. It’s important to weigh the complexity of integration against the potential benefits, ensuring that the improved performance justifies the effort and cost involved.
3. NVIDIA H100 Dual-GPU: Overview
Architecture & Specifications
When you consider the H100 for your dual-GPU setup, you’re looking at a GPU that represents the pinnacle of NVIDIA’s Hopper architecture. Built using TSMC’s 4N process technology, the H100 packs 80 billion transistors into a single GPU, making it a powerhouse for AI workloads. This GPU is designed to accelerate large-scale AI tasks, providing a balanced blend of performance and efficiency for enterprises seeking a high-performing, yet budget-conscious solution.
Key features of the H100 include:
- Fourth-Gen Tensor Cores: These cores are optimized for FP8, FP16, and TF32 precision, providing up to three times the matrix operation speed of previous architectures like the A100.
- HBM3 Memory: With 80GB of high-bandwidth memory per GPU, delivering 3.35 TB/s of memory bandwidth, the H100 can easily handle large datasets and intricate model parameters, making it ideal for tasks like training neural networks or processing large-scale LLMs.
- NVLink 4.0: The H100 supports bidirectional bandwidth of 900 GB/s between dual GPUs. This ensures that communication between GPUs remains fast, reducing bottlenecks that could slow down multi-GPU setups.
- PCIe Gen5 Support: This latest PCIe standard offers double the data transfer speeds of Gen4, enabling smooth integration with your existing server infrastructure and enhancing overall system efficiency.
Performance Benchmarks
The H100 truly shines when it comes to performance across both training and inference tasks:
- Training Speed: The H100 trains models like ResNet-50 in under six minutes (1,000 epochs). Its training of GPT-3-sized models is three times faster than its predecessor, the A100, thanks to optimizations like sparsity acceleration and the transformer engine.
- Inference Throughput: The H100 delivers up to 30 times the throughput of the A100 for large language models, handling over 4,000 tokens per second in real-time inference scenarios.
- Scalability: When you scale the H100 to a dual-GPU setup, the performance increases almost linearly. This makes the H100 ideal for distributed training and real-time AI solutions where time-to-market is critical.
Power Efficiency
The H100 isn’t just about raw power—it’s about delivering efficient performance:
- Thermal Design Power (TDP): At 700W per GPU, the H100 SXM5 variant demands robust cooling solutions. Depending on your infrastructure, you may need to implement liquid cooling or advanced airflow systems in your data center.
- Performance per Watt: While the H100 has a relatively high power draw, the Hopper architecture provides significant energy efficiency. It delivers up to 2x better performance per watt compared to the A100, making it a sustainable option for enterprises that need both power and efficiency.
Cost Analysis
For many enterprises, cost is a decisive factor, and the H100’s price reflects its position as a balance between performance and affordability:
- Hardware Costs: A single H100 GPU typically costs between $30,000 and $40,000. A dual-GPU setup would naturally double this price, adding further costs for NVLink bridges and cooling infrastructure.
- Licensing: Enterprises deploying the H100 for AI workloads will likely need to invest in the NVIDIA AI Enterprise Suite, which can cost around $10,000 per year per GPU for optimized software support.
- Total Ownership: Despite the high initial investment, the H100’s efficiency and speed reduce long-term operational costs. Faster training cycles reduce cloud compute expenses, and the lower idle times improve overall resource utilization, which can help offset the higher upfront costs.
Ideal Use Cases
The H100 Dual-GPU is a solid choice if you’re balancing performance with budget:
- Traditional AI Workloads: For tasks like convolutional neural networks (CNNs) for image recognition, recurrent neural networks (RNNs) for time-series analysis, or smaller-scale LLMs (under 50B parameters), the H100 excels without overkill.
- Budget-Conscious Scaling: If your goal is to scale AI infrastructure without splurging on cutting-edge architectures, the H100 is an excellent choice.
- Hybrid Cloud Deployments: The H100 fits well in hybrid setups where power efficiency and space are more critical than bleeding-edge performance.
- Industries: The H100 is widely used in sectors like healthcare (for medical imaging), finance (for fraud detection), and manufacturing (for predictive maintenance), where robust, efficient AI solutions are essential without requiring the absolute latest technology.
4. NVIDIA H200 NVL Dual-GPU: Overview
Architecture & Specifications
The NVIDIA H200 NVL, an evolution of the Hopper architecture, is engineered for enterprises like yours that are pushing the boundaries of AI capabilities. Built to handle the most demanding workloads, the H200 NVL offers improvements that ensure your infrastructure stays ahead of the curve. With advancements in memory and interconnect technologies, the H200 NVL is designed to meet the needs of next-gen AI models, including those with trillion-parameter architectures.
Key features of the H200 NVL include:
- HBM3e Memory: With 141GB of HBM3e memory per GPU and an impressive 4.8 TB/s memory bandwidth, the H200 NVL surpasses the H100 in handling massive datasets and intricate AI models. This ensures you’re not limited by memory bottlenecks, allowing for smooth, uninterrupted training and inference even with the largest models.
- Fifth-Gen NVLink: The H200 NVL integrates fifth-generation NVLink, delivering 1.8 TB/s bidirectional bandwidth between GPUs—double the bandwidth of the H100. This reduces latency and enhances multi-GPU communication, ensuring that your GPUs work efficiently together to handle complex AI workloads.
- Transformer Engine v2: Optimized for FP8 and FP6 precision, this upgrade accelerates training and inference for large language models while maintaining accuracy, further improving throughput for AI tasks that require massive computation.
- PCIe Gen5 x16 Support: Ensuring rapid data transfer, PCIe Gen5 x16 support makes the H200 NVL ideal for real-time AI applications, allowing data to flow freely between the GPUs and host systems.
Performance Benchmarks
The H200 NVL is designed to take on the heaviest AI tasks with ease:
- LLM Training: When training models like GPT-4, the H200 NVL cuts training times by 40% compared to the H100, transforming what could be weeks of work into mere days. This is critical for enterprises like yours that need to stay agile and ahead of the competition.
- Inference Dominance: In real-time inference, the H200 NVL achieves 2.5x higher throughput than the H100, processing over 10,000 tokens per second. This makes it ideal for use cases where millisecond latency is crucial, such as chatbots or recommendation systems.
- Memory Bandwidth Advantage: With 4.8 TB/s of memory bandwidth, the H200 NVL excels in memory-bound tasks like fine-tuning large models. Its memory architecture reduces data fetch delays by 30% compared to the H100, making it the better option for workloads that require high bandwidth.
Power Efficiency
While the H200 NVL demands more power, its improved architectural efficiency ensures it’s still a sustainable option:
- TDP: The H200 NVL has a thermal design power (TDP) of 600W per GPU, requiring advanced cooling solutions such as direct-to-chip liquid cooling. While this is a step up from the H100, the improved performance justifies the added energy costs for enterprises running intensive AI workloads.
- Efficiency Gains: Despite its higher power draw, the H200 NVL delivers 1.5x better performance per watt compared to the H100 in large language model workloads. For businesses with heavy AI demands, this efficiency can result in significant long-term energy savings, despite the higher operational costs.
Cost Analysis
The H200 NVL presents a higher upfront investment, but it provides value in the form of accelerated performance and longer-term savings:
- Hardware Costs: At $40,000 to $50,000 per GPU, a dual-H200 setup starts at around $100,000, excluding costs for NVLink bridges and compatible servers. This makes the H200 NVL a premium choice compared to the H100.
- Operational Costs: While the H200 NVL demands more energy and cooling, the savings in time—such as reducing training time from 14 days to 10—can lead to a 30% reduction in cloud computing costs over time. For large-scale enterprises, these savings can quickly offset the higher initial investment.
- Licensing: As with the H100, the H200 NVL requires the NVIDIA AI Enterprise Suite. However, for specialized workloads like LLM optimization, you may need additional software or libraries, such as NeMo, to fully unlock its potential.
Ideal Use Cases
The H200 NVL is built for enterprises with large-scale, cutting-edge AI needs:
- Cutting-Edge LLMs: If your business is training trillion-parameter models like GPT-4 or Claude, the H200 NVL is designed to handle those workloads without the memory constraints that would slow down the H100.
- Real-Time Inference: For mission-critical applications, such as autonomous vehicles or fraud detection, where milliseconds matter, the H200 NVL’s 2.5x faster inference throughput will deliver the low-latency performance you need to stay competitive.
- Future-Proofing: The H200 NVL is designed with future AI advancements in mind, supporting models that exceed 100B parameters and ensuring that your infrastructure remains relevant for 4 to 5 years—avoiding the need for near-term upgrades.
5. Head-to-Head Comparison
Performance
When comparing the H100 and H200 NVL, the most important factor for your enterprise is how each GPU performs relative to its cost. Here’s a breakdown:
- Training Speed Per Dollar:
-
- H100: If cost-efficiency is your primary concern, the H100 offers excellent value. It trains models like GPT-3 at approximately $0.25 per epoch, factoring in both hardware and energy costs. This makes it a solid choice for enterprises looking to maximize the value they get from each dollar spent.
- H200 NVL: The H200 NVL, while around 30% more expensive, trains the same models 40% faster. This reduces the cost per epoch to roughly $0.22 in the long term. If you’re running high-volume workloads, the H200 NVL can save you considerable time and money, especially in large-scale AI training.
- Memory Capacity & Bandwidth:
-
- H100: The H100 features 80GB of HBM3 memory with a bandwidth of 3.35 TB/s. This is more than enough for models with up to 50 billion parameters, making it a reliable choice for many AI applications.
- H200 NVL: With 141GB of HBM3e memory and a bandwidth of 4.8 TB/s, the H200 NVL can easily support trillion-parameter models, reducing memory bottlenecks by 30%. If your enterprise plans to work with the next generation of LLMs, the H200 NVL’s superior memory performance will pay off.
Cost
-
- H100: A dual-H100 setup typically costs around $70,000, making it an affordable option for mid-sized enterprises or departments with tighter budgets. It offers an accessible entry point for those seeking solid performance without an excessive upfront commitment.
- H200 NVL: A dual-H200 NVL setup costs around $100,000, which is a significant premium. This price reflects the H200’s advanced features and scalability, making it an investment for enterprises that need cutting-edge performance to handle the most demanding AI workloads.
-
- H100: The H100 may require more cloud resources over time due to slower training cycles. For example, a 14-day LLM training cycle could cost around $12,000. If you’re training multiple models, these costs can quickly add up.
- H200 NVL: By completing the same tasks 40% faster, the H200 NVL reduces training time from 14 days to 10. This results in a savings of about $3,600 in cloud fees. While energy costs rise by about 20%, the time saved on training cycles can still justify the higher investment, particularly for enterprises with heavy, ongoing AI needs.
Scalability
-
- Both: Both the H100 and H200 NVL scale efficiently across NVIDIA’s DGX SuperPOD, supporting up to 1000+ nodes. However, the H200 NVL’s 1.8 TB/s NVLink bandwidth provides a clear advantage in reducing inter-node latency, which is crucial when training massive models across multiple nodes.
- H100: The H100, with lower bandwidth (900 GB/s), can face challenges when scaling beyond 500 nodes. This may lead to inefficiencies, especially for large-scale distributed training where GPU utilization is critical.
NVLink Scalability:
-
- H200 NVL: The H200 NVL supports up to 18 GPUs in a single NVLink domain, compared to the H100’s 8. This allows you to build denser clusters with fewer communication hops, enhancing overall performance and scalability for large AI projects.
Software & Ecosystem
-
- Both: Both GPUs support CUDA 12+, PyTorch, and TensorFlow. However, the H200 NVL unlocks exclusive features like FP6 precision for TensorRT-LLM, optimizing inference speed and reducing computational overhead.
- H100: While the H100 supports these frameworks, it lacks native support for newer techniques like 4-bit floating-point quantization, which can limit its future-proofing capabilities as AI workloads evolve.
Vendor Support:
-
- H200 NVL: If you choose the H200 NVL, you’ll benefit from priority access to NVIDIA’s AI Enterprise Suite updates, including early beta tools for LLM optimization. This ensures that your business remains on the cutting edge of AI advancements.
- H100: The H100 also benefits from NVIDIA’s support but does not offer the same level of access to upcoming features, making it a less flexible option for enterprises aiming to remain at the forefront of AI innovation.
Compatibility
-
- H100: The H100 is compatible with most existing PCIe Gen5 servers, and its 700W TDP is manageable with standard air cooling solutions. This makes it a smoother fit for enterprises looking to avoid significant upgrades to their data center infrastructure.
- H200 NVL: The H200 NVL requires advanced cooling systems such as direct-to-chip liquid cooling, which adds an additional $15,000 for legacy data centers. For enterprises with existing infrastructure, this may necessitate costly upgrades but ensures better thermal efficiency for high-performance AI workloads.
- H100: The H100 integrates easily with older NVIDIA GPUs, such as A100 clusters, offering a simpler upgrade path for businesses with existing GPU infrastructure.
- H200 NVL: The H200 NVL, however, requires full-stack upgrades to Gen5 servers, limiting its backward compatibility with legacy systems. This might be a consideration if your infrastructure relies heavily on older hardware.
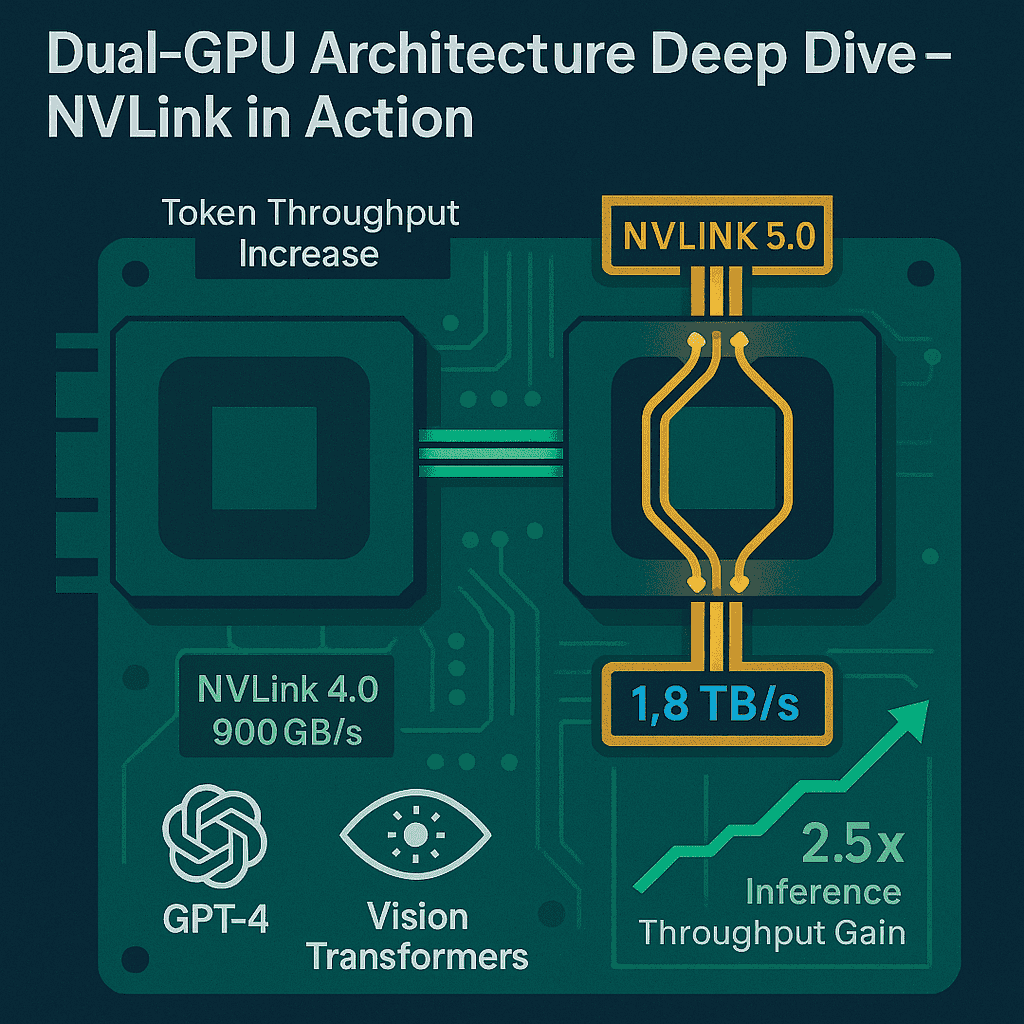
6. ROI Analysis for Enterprise AI
Calculating ROI
When evaluating ROI in AI infrastructure, there are three core pillars that will influence your decision: time-to-insight, operational efficiency, and model accuracy. These factors will not only impact your bottom line but will also shape your ability to deploy AI models quickly and cost-effectively.
- Time-to-Insight: Faster training cycles mean quicker deployment of AI models that can drive business value. For instance, by reducing the time it takes to train models, you can launch new features faster, gaining a competitive edge in your market. For you, the H200 NVL can reduce training cycles by as much as 40%, speeding up your time-to-market and accelerating your return on investment.
- Operational Efficiency: With dual-GPU setups, you’ll experience improved resource utilization, reduced idle times, and greater overall system efficiency. The H200 NVL’s ability to process data faster and with less latency can significantly lower your operational costs, especially when working on high-demand workloads that would otherwise require more time and energy.
- Model Accuracy: The H200 NVL’s superior memory bandwidth and precision (FP8) can improve the accuracy of your models, reducing the likelihood of errors in production. More accurate models lead to better decision-making, which translates into a higher ROI over time by minimizing costly mistakes in areas like fraud detection, predictive maintenance, or recommendation systems.
H100 ROI Scenario
- Lower Upfront Costs: The H100’s dual-GPU setup costs around $70,000, making it an attractive option for enterprises with more constrained budgets. It’s a solid choice if your immediate goal is to scale up your AI capabilities without a hefty initial outlay.
- Moderate Power Use: At 700W per GPU, the H100 requires about $3,000 per month for energy and cooling for a 10-node cluster. While not insignificant, these costs are manageable for many enterprises.
- Shorter Lifecycle: The H100 may require an upgrade sooner than the H200 NVL, especially as AI models grow in size and complexity. For enterprises training models with over 50 billion parameters, the H100 may start to show its limitations in just a few years. However, if you’re focused on shorter-term projects with less demanding workloads, the H100’s ROI can be significant.
- ROI Sweet Spot: The H100 shines for enterprises prioritizing immediate gains. For instance, a healthcare startup training 10B-parameter models for medical imaging could yield $500K in annual efficiency gains by improving diagnostic speed and accuracy.
H200 NVL ROI Scenario
- Higher Initial Spend: The H200 NVL’s dual-GPU setup comes with a price tag of around $100,000, which includes the GPUs, NVLink bridges, and necessary cooling upgrades. This higher upfront investment is a consideration, but it’s a cost you can justify if your enterprise is looking for a long-term solution with the ability to scale.
- Reduced Latency: The H200 NVL’s 2.5x faster inference processing means reduced latency, critical for applications where time is money—think real-time fraud detection or autonomous vehicles. In sectors where even milliseconds matter, the ROI can be substantial. For instance, in stock trading, the H200’s faster processing could reduce decision latency by 1.5ms, translating into millions in annual revenue gains.
- Longer Relevance: The H200 NVL’s memory architecture and NVLink scalability extend its useful lifespan to 4-5 years, even as AI models continue to grow in size. This reduces the need for frequent hardware refreshes, making the H200 a future-proof solution that protects your investment.
- ROI Sweet Spot: For enterprises like yours, the H200 NVL is ideal if you need to handle complex, large-scale AI workloads—such as training trillion-parameter models (e.g., GPT-4). A tech giant training GPT-5, for example, could save $1M/year in cloud costs by cutting training time from 60 to 40 days. Over a 5-year span, the premium for the H200 NVL pays for itself tenfold.
Break-Even Analysis: When Does the H200 Justify Its Cost?
The H200 NVL’s ROI surpasses the H100’s when:
- Workloads Demand Speed: If time is a direct contributor to revenue—such as for AI-first companies releasing features quarterly—the H200’s 40% faster training time makes it a worthwhile investment. In such cases, the H200 pays for itself within months, speeding up time-to-market and delivering quicker returns.
- Scale Exceeds 50B Parameters: For cutting-edge models like GPT-4 (1.7 trillion parameters), the H100’s memory limitations can lead to additional overhead, such as the need for model parallelism. These workarounds increase costs by about 20%. The H200 NVL, with its larger memory and improved scalability, eliminates these bottlenecks, justifying its higher cost much sooner.
- Latency = Revenue: In real-time applications—such as personalized ad targeting—the H200 NVL’s 2.5x faster inference throughput could increase ad revenue by 15%. This type of performance gain could justify the additional premium in just 6–8 months.
Final Takeaway
- H100: The H100 delivers the best ROI for enterprises dealing with traditional workloads and short-term projects. If your business focuses on scaling AI within budget constraints, the H100 is a practical, cost-effective choice.
- H200 NVL: If you’re aiming for long-term AI leadership with high-demand workloads—like training trillion-parameter models or minimizing latency in real-time systems—the H200 NVL provides unmatched value. Its higher upfront cost is a strategic investment that ensures your enterprise stays ahead in the rapidly evolving AI landscape.
This analysis arms you with the insights needed to evaluate your AI infrastructure needs, helping you decide whether the H100 or H200 NVL aligns better with your business’s strategic goals.
7. Use Case Recommendations
Choose the NVIDIA H100 Dual-GPU if:
- Budget Constraints are Critical
If your enterprise has a limited budget but still needs to scale its AI capabilities, the H100 is an excellent choice. Its dual-GPU setup, starting at around $70,000 (hardware only), makes it one of the more affordable options for businesses looking to optimize their AI infrastructure without significant upfront investments. The H100 delivers solid performance and efficiency without the premium cost of newer architectures like the H200 NVL.
For example, a retail company deploying recommendation engines or a university lab training models for climate prediction could benefit from the H100. With moderate power consumption (700W per GPU), its total cost of ownership (TCO) remains manageable for mid-sized businesses and startups looking for a balance between performance and cost.
- Workloads Prioritize Moderate-Scale Models
If your business is focused on smaller-scale AI models—like convolutional neural networks (CNNs) for image recognition or recurrent neural networks (RNNs) for time-series forecasting—the H100 is more than capable. Its 80GB HBM3 memory and 3.35 TB/s memory bandwidth are well-suited for models with fewer than 50 billion parameters.
For instance, a healthcare provider running ResNet-50 for MRI analysis or a fintech firm fine-tuning a BERT-based fraud detection system can achieve excellent results with the H100 without overprovisioning. These models don’t require the immense memory and bandwidth of the H200 NVL, making the H100 a more cost-effective solution.
- Existing Infrastructure Favors Lower TDP
If your enterprise has an existing infrastructure designed for lower TDP GPUs (like older A100 clusters), the H100’s 700W TDP will integrate seamlessly with your current systems. The H100 works well with standard air-cooled data centers, allowing you to avoid the need for costly upgrades or retrofitting.
For industries like manufacturing or logistics—where retrofitting facilities could disrupt operations—the H100 offers an efficient, low-risk upgrade path without requiring significant changes to your existing infrastructure.
Choose the NVIDIA H200 NVL Dual-GPU if:
- Running State-of-the-Art LLMs (e.g., GPT-4, Claude)
If your enterprise is focused on cutting-edge LLMs with trillions of parameters, the H200 NVL is the clear choice. Its 141GB HBM3e memory and 4.8 TB/s bandwidth allow it to handle memory-intensive tasks with ease, reducing bottlenecks and improving overall training and inference performance.
For example, if you’re building a 1.7 trillion-parameter model for legal document automation, the H200 NVL could reduce training time from 30 to 18 days, accelerating your time-to-market. The H200 NVL is the optimal choice when dealing with the most complex models that require immense computational power.
- Minimizing Inference Latency is Paramount
If real-time decision-making is critical for your enterprise—such as autonomous vehicle systems, high-frequency trading, or live customer service chatbots—the H200 NVL will help you meet these demanding latency requirements. With its 2.5x faster inference throughput, processing over 10,000 tokens per second, the H200 NVL ensures low-latency, high-performance results under heavy loads.
A stock trading firm, for example, could leverage the H200 NVL to make AI-driven trades 1.5ms faster, potentially translating that small gain into millions of dollars in annual revenue. In sectors where every millisecond counts, the H200 NVL’s speed delivers a clear competitive advantage.
- Future-Proofing for Next-Gen AI Models
If your enterprise is preparing for the future of AI—anticipating rapid model growth, new AI techniques, and evolving workloads—the H200 NVL is designed to handle these changes. With its fifth-gen NVLink, FP6 precision, and support for large-scale models (100B+ parameters), the H200 NVL provides a longer lifespan (4-5 years) compared to the H100.
This makes the H200 NVL the better choice for businesses focused on long-term scalability. For example, a defense contractor developing AI for real-time battlefield simulations or a cloud provider offering AI-as-a-service (AIaaS) could benefit from the H200 NVL’s robust architecture, ensuring their infrastructure remains capable as models and workloads grow.
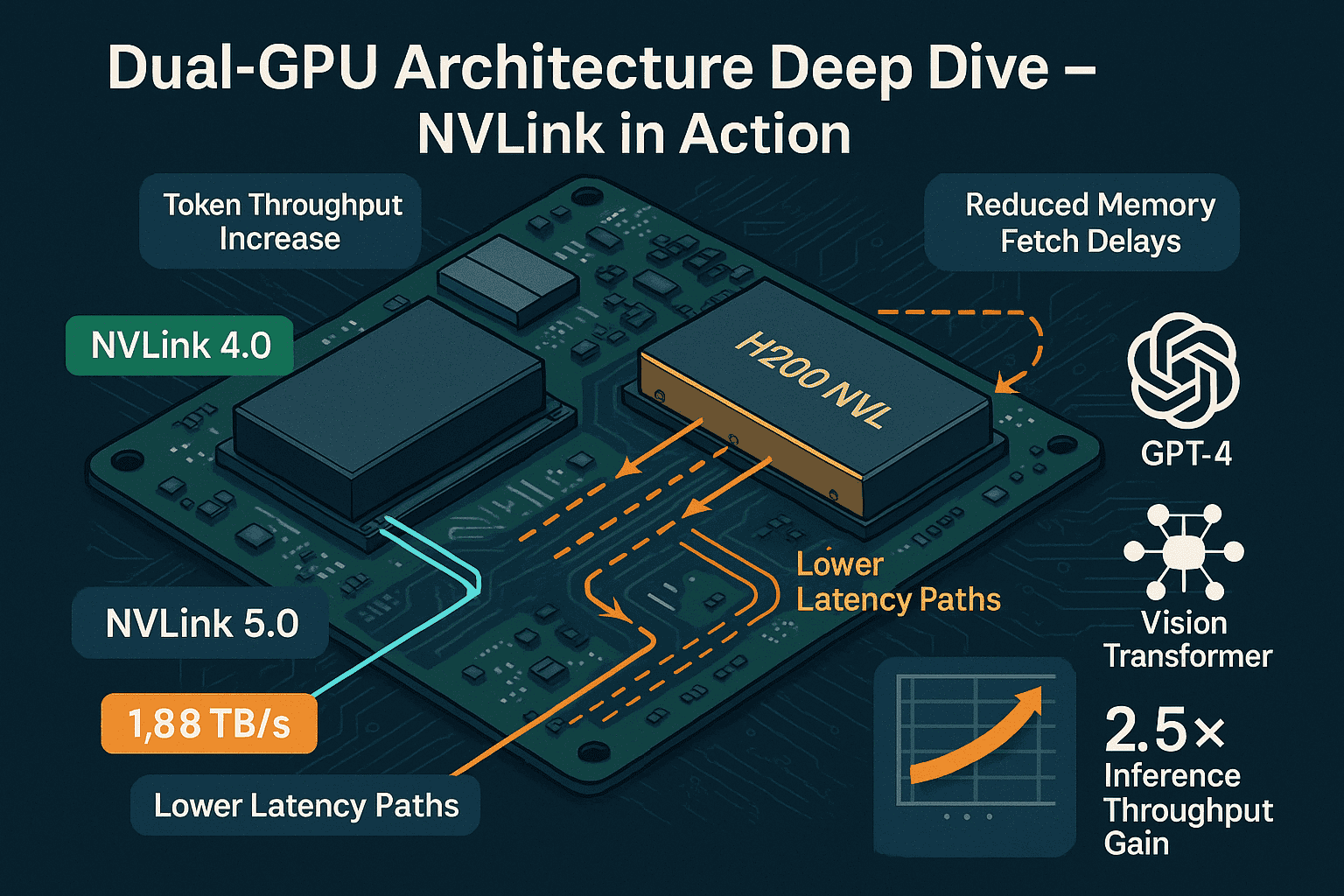
Industry-Specific Guidance
- Healthcare (H100): If your focus is on medical imaging analysis or smaller AI models for drug discovery, the H100 is well-suited to these tasks. Its affordability and performance make it ideal for hospitals, research labs, and healthcare startups that need reliable AI infrastructure without breaking the bank.
- Finance (H200 NVL): For high-frequency trading, algorithmic fraud detection, or personalized banking chatbots, the H200 NVL is the optimal choice. Its low-latency, high-throughput capabilities ensure that your AI models operate in real-time, giving you a crucial edge in the fast-paced financial sector.
- Automotive (H200 NVL): In the automotive industry, especially in autonomous vehicle systems, the H200 NVL’s speed and memory bandwidth provide the computational power needed for complex perception systems and real-time decision-making. The H200 NVL helps ensure your vehicles can process data quickly and accurately, even in challenging environments.
- Academia (H100): Universities and research institutions working on moderate-scale AI projects, such as training models for climate prediction or small-scale natural language processing, will find the H100 to be a cost-effective, powerful option. It balances performance and affordability, making it ideal for academic research and student-led AI initiatives.
8. Conclusion
The decision between the H100 and H200 NVL boils down to your enterprise’s current needs and long-term AI strategy. If you’re looking for a cost-effective solution that handles traditional AI workloads without a significant upfront investment, the H100 offers a strong return on investment. It provides reliable performance for a wide range of tasks, from image recognition to time-series forecasting, making it an ideal choice for enterprises that need to balance performance and budget.
On the other hand, if your business is aiming for AI leadership, where speed, scalability, and future-proofing are paramount, the H200 NVL offers unmatched value. With its advanced memory architecture, faster inference processing, and ability to handle trillion-parameter models, the H200 NVL is built for enterprises that need to stay ahead in a rapidly evolving AI landscape. While the H200 NVL comes with a higher initial cost, the long-term benefits—especially for high-demand, real-time applications and cutting-edge LLMs—make it a strategic investment in your AI infrastructure.
By understanding the strengths of each GPU and evaluating your enterprise’s AI goals, you can make a more informed decision that aligns with both your short-term needs and long-term vision. Whether you choose the reliable performance and affordability of the H100 or the advanced, future-proof capabilities of the H200 NVL, both GPUs represent a solid foundation for building your AI infrastructure and driving innovation.
In the race to harness AI’s full potential, the right GPU is more than just hardware—it’s a strategic asset that will impact your business for years to come.
9. Final Recommendations
In the decision-making process between the H100 and H200 NVL, it’s essential to assess both your immediate needs and your longer-term goals. Here’s a final breakdown to guide your decision:
Choose the H100 if:
-
- You need a cost-effective solution that delivers strong, reliable performance for a wide range of AI tasks.
- Your enterprise is looking to scale efficiently without large upfront costs.
- You’re working with moderate-scale AI models (under 50B parameters) and need a system that can handle traditional AI workloads like CNNs, RNNs, and smaller language models.
- You’re operating in an existing infrastructure where power consumption and cooling requirements are crucial for keeping operational costs low.
Choose the H200 NVL if:
-
- Your enterprise is committed to scaling with the next generation of AI, handling complex, trillion-parameter models, and optimizing real-time inference.
- You need to future-proof your infrastructure with the ability to tackle next-gen AI workloads, such as GPT-4 or multimodal systems.
- Low latency and high throughput are critical to your operations, especially in industries like autonomous vehicles, finance, and real-time AI-driven services.
- You’re willing to make a higher initial investment for long-term savings, including cutting training cycles, reducing cloud compute costs, and minimizing upgrade cycles for the next 4–5 years.
Both GPUs have their merits, depending on your enterprise’s AI strategy and growth trajectory. If you’re looking for a workhorse that’s reliable and well-suited to most tasks, the H100 is a great choice. However, if you are building infrastructure for tomorrow’s AI challenges, the H200 NVL stands as the definitive option.
Ultimately, both GPUs will position you to drive AI innovation within your enterprise. The choice is about aligning your investment with your business’s unique needs, whether that means optimizing for cost today or investing in long-term capability for the future.